Planet Python
Last update: July 01, 2025 09:42 PM UTC
July 01, 2025
PyCoder’s Weekly
Issue #688: Checking Dicts, DuckDB, Reading shelve.py, and More (July 1, 2025)
Real Python
Implementing the Factory Method Pattern in Python
Learn how to use the Factory Method pattern in Python, when to apply it, how to refactor your code for it, and explore a reusable implementation.
Mike Driscoll
Creating a Website with Sphinx and Markdown
Sphinx is a Python-based documentation builder. The Python documentation is written using Sphinx. The Sphinx project supports using ReStructuredText and Markdown, or a mixture of the two. Each page of your documentation or website must be written using one of those two formats. The original Python 101 website uses an old version of Sphinx, for […]
The post Creating a Website with Sphinx and Markdown appeared first on Mouse Vs Python.
Zero to Mastery
[June 2025] Python Monthly Newsletter 🐍
67th issue of Andrei Neagoie's must-read monthly Python Newsletter: Fastest Python, MCP Eats The World, Optimize Your Python, and much more. Read the full newsletter to get up-to-date with everything you need to know from last month.
Tryton News
Newsletter July 2025
In the last month we focused on fixing bugs, improving the behaviour of things, speeding-up performance issues - building on the changes from our last release. We also added some new features which we would like to introduce to you in this newsletter.
For an in depth overview of the Tryton issues please take a look at our issue tracker or see the issues and merge requests filtered by label.
Changes for the User
Accounting, Invoicing and Payments
Now we provide a dedicated list view to display the children lines on budget forms.
As we do not need the full record name but just the current name when editing a child from its parent form.
User Interface
We now converted Unidentified Image Errors into more user friendly validation errors. The normalisation/preprocess of images using PIL (Python Image Library) may raise these exceptions.
Now we keep selected rows on xxx2Many
widget on reload.
We now limit the height of the completion drop downs and the actions are set sticky to be always visible.
System Data and Configuration
In order to have always the same results no matter of the order of the lines, we now round the tax amount of each line before adding it to the total tax.
In the past we rounded the tax amount per line after it was added to the total tax. With the used bankers rounding the issue is that when the result is like .xy5
(if rounding precision is set to 2 digits) it may be rounded up or down depending if the y
-digit is even or odd.
New Releases
We released bug fixes for the currently maintained long term support series
7.0 and 6.0, and for the penultimate series 7.6, 7.4 and 7.2.
1 post - 1 participant
Seth Michael Larson
Hand-drawn QR codes
June 30, 2025
Real Python
Use TorchAudio to Prepare Audio Data for Deep Learning
Learn to prepare audio data for deep learning in Python using TorchAudio. Explore how to load, process, and convert speech to spectrograms with PyTorch tools.
Quiz: Use TorchAudio to Prepare Audio Data for Deep Learning
Test your grasp of audio fundamentals and working with TorchAudio in Python! You'll cover loading audio datasets, transforms, and more.
Django Weblog
Our 2024 Annual Impact Report
Django has always been more than just a web framework; it’s a testament to what a dedicated community can build together. Behind every Django release, bug fix, or DjangoCon is a diverse network of people working steadily to strengthen our open-source ecosystem. To celebrate our collective effort, the Django Software Foundation (DSF) is excited to share our 2024 Annual Impact Report 🎉
In this report, you’ll discover key milestones, narratives of community folks, the impact of the events running throughout the year, and much more, ramping up to how we’re laying the groundwork for an even more resilient and inclusive Django community.
Why we publish this report
Transparency is essential for our community-driven organization. Everyone deserves to know how our work and investments translate into real impact. It’s more than just statistics. It’s our way to:
- Show how your contributions make a difference, with vibrant highlights from the past year.
- Reflect on community progress, recognizing the people and ideas that keep Django thriving.
- Invite more individuals and organizations to get involved.
Looking ahead: call to action
As we make progress through 2025, the Django Software Foundation remains dedicated to strengthening the ecosystem that supports developers, contributors, and users around the world. With a growing network of working groups, community initiatives, and the commitment of volunteers, we’re focused on nurturing the people and executing ideas that make Django what it is: the web framework for perfectionists with deadlines.
Help keep this momentum strong by supporting Django through any of the following ways:
- Donate to Django to support future development
- Convince your company to become a Corporate Member
- Join the Foundation as an Individual Member
- Get involved with the working groups
- Join our community on the Django Forum or Discord server.
- Follow and re-share our posts on Mastodon, on Bluesky, or on X.
- Follow our page on LinkedIn.
Thank you, everyone, for your dedication and efforts. Here’s to another year of collaboration, contribution, and shared success!
Python Bytes
#438 Motivation time
Topics include Python Cheat Sheets from Trey Hunner, Automatisch, mureq-typed, and My CLI World.
June 27, 2025
Hugo van Kemenade
Run coverage on tests
I recommend running coverage on your tests.
Here’s a couple of reasons why, from the past couple of months.
Example one #
When writing tests, it’s common to copy and paste test functions, but sometimes you forget to rename the new one (see also: the Last Line Effect).
For example:
def test_get_install_to_run_with_platform(patched_installs):
i = installs.get_install_to_run("<none>", None, "1.0-32")
assert i["id"] == "PythonCore-1.0-32"
assert i["executable"].match("python.exe")
i = installs.get_install_to_run("<none>", None, "2.0-arm64")
assert i["id"] == "PythonCore-2.0-arm64"
assert i["executable"].match("python.exe")
def test_get_install_to_run_with_platform(patched_installs):
i = installs.get_install_to_run("<none>", None, "1.0-32", windowed=True)
assert i["id"] == "PythonCore-1.0-32"
assert i["executable"].match("pythonw.exe")
i = installs.get_install_to_run("<none>", None, "2.0-arm64", windowed=True)
assert i["id"] == "PythonCore-2.0-arm64"
assert i["executable"].match("pythonw.exe")
The tests pass, but the first one is never run because its name is redefined. This clearly shows up as a non-run test in the coverage report. In this case, we only need to rename one of them, and both are covered and pass.
But sometimes there’s a bug in the test which would cause it to fail, but we just don’t know because it’s not run.
Example two #
This is more subtle:
im = Image.new("RGB", (1, 1))
for colors in (("#f00",), ("#f00", "#0f0")):
append_images = (Image.new("RGB", (1, 1), color) for color in colors)
im_reloaded = roundtrip(im, save_all=True, append_images=append_images)
assert_image_equal(im, im_reloaded)
assert isinstance(im_reloaded, MpoImagePlugin.MpoImageFile)
assert im_reloaded.mpinfo is not None
assert im_reloaded.mpinfo[45056] == b"0100"
for im_expected in append_images:
im_reloaded.seek(im_reloaded.tell() + 1)
assert_image_similar(im_reloaded, im_expected, 1)
It’s not so obvious when looking at the code, but Codecov highlights a problem:
The append_images
generator is being consumed inside roundtrip()
, so we have nothing
to iterate over in the for
loop – hence no coverage. The
fix is to
use a list instead of a generator.
Header photo: Misplaced manhole cover (CC BY-NC-SA 2.0 Hugo van Kemenade).
Real Python
The Real Python Podcast – Episode #255: Structuring Python Scripts & Exciting Non-LLM Software Trends
What goes into crafting an effective Python script? How do you organize your code, manage dependencies with PEP 723, and handle command-line arguments for the best results? Christopher Trudeau is back on the show this week, bringing another batch of PyCoder's Weekly articles and projects.
Luke Plant
Statically checking Python dicts for completeness
A Pythonic way to ensure that your statically-defined dicts are complete, with full source code.
PyPodcats
Episode 9: With Tamara Atanasoska
Learn about Tamara's journey. Tamara has been contributing to open source projects since 2012. She participated in Google Summer of Code to contribute to projects like Gnome and e-cidadania.
Django Weblog
Watch the DjangoCon Europe 2025 talks
They’re now all available to watch on YouTube, with a dedicated playlist ⭐️ DjangoCon Europe 2025 Dublin. For more quality Django talks in 2025, check out our next upcoming events!
All the DjangoCon Europe talks
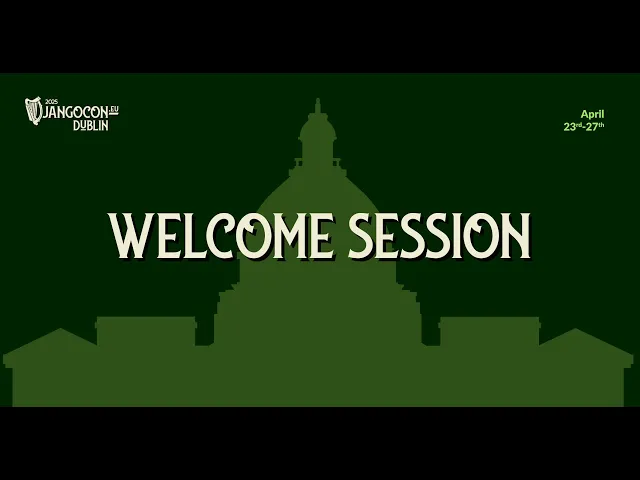
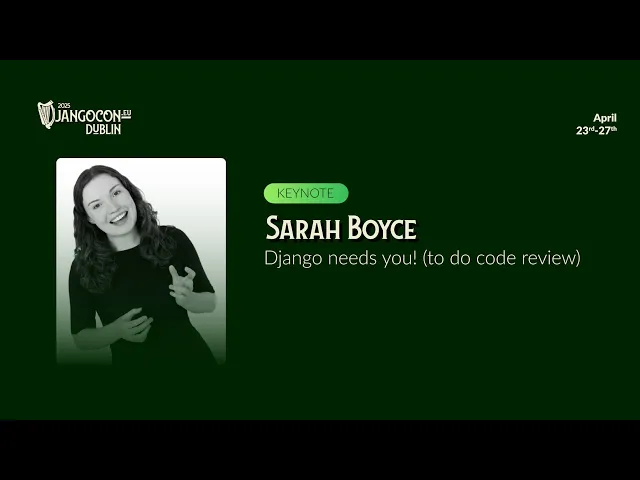
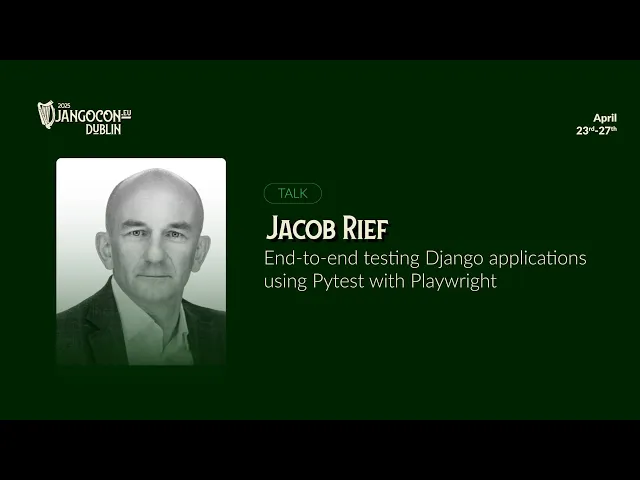
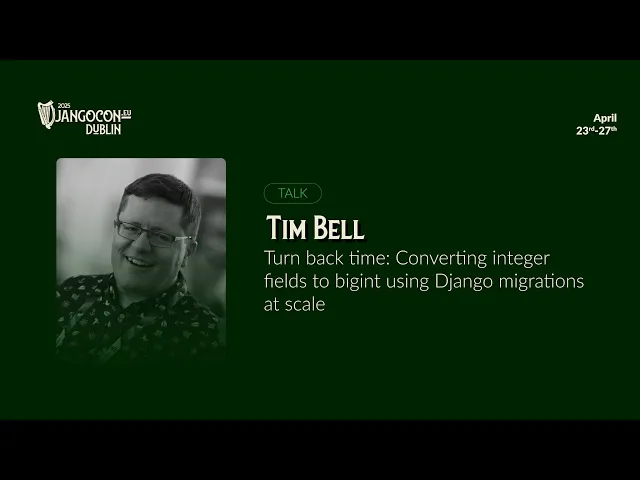
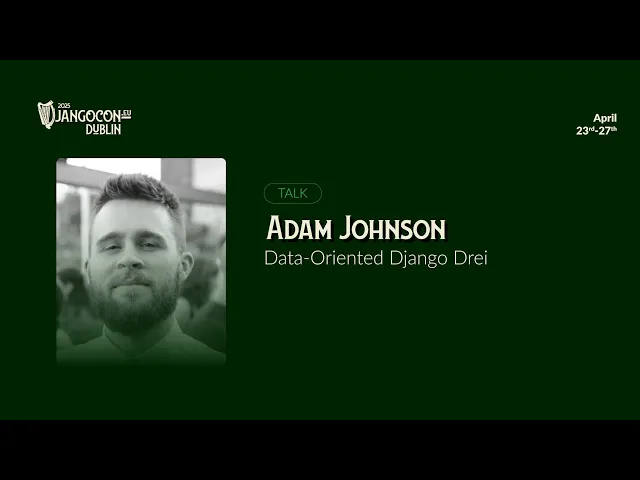
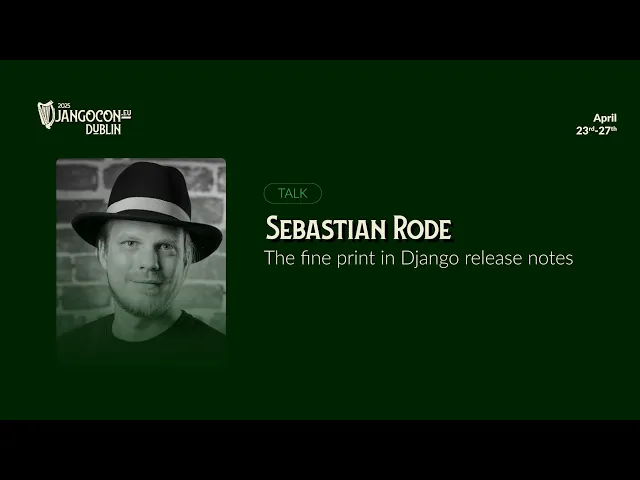
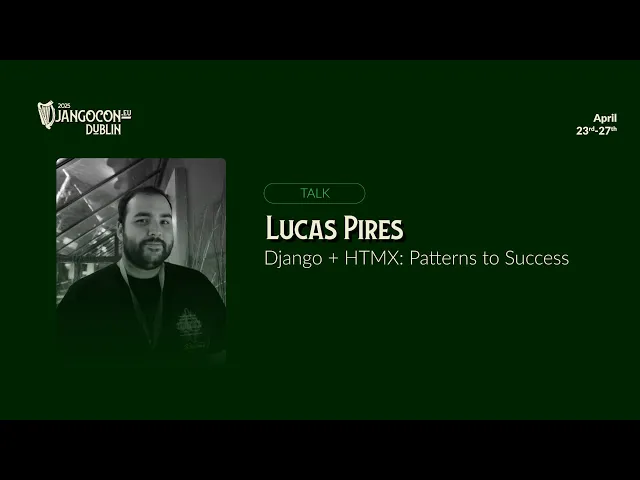
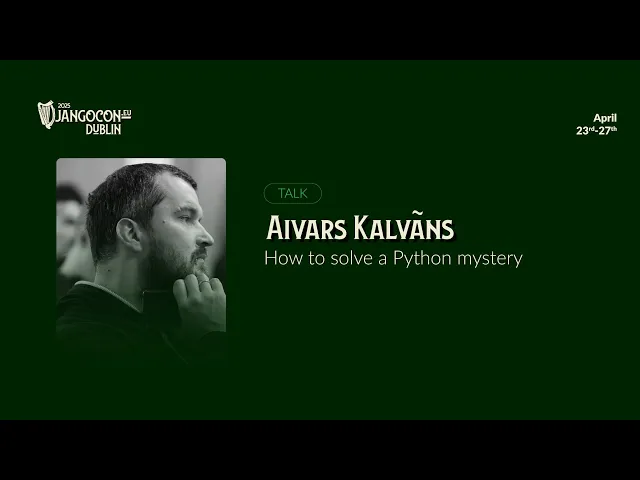
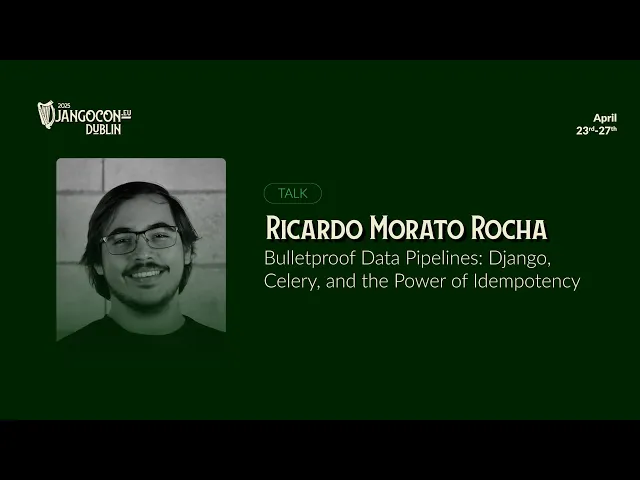
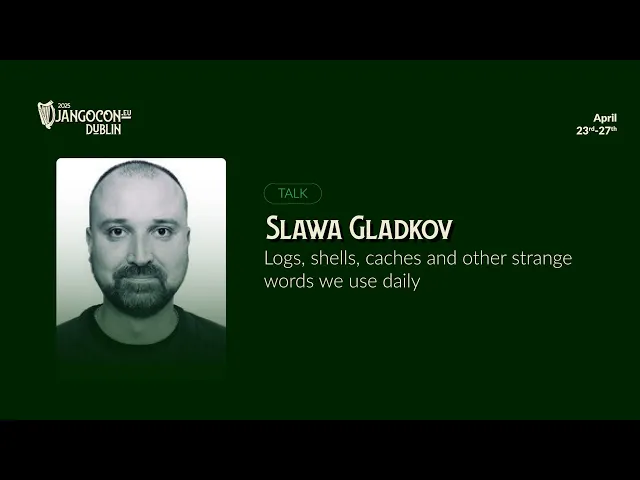
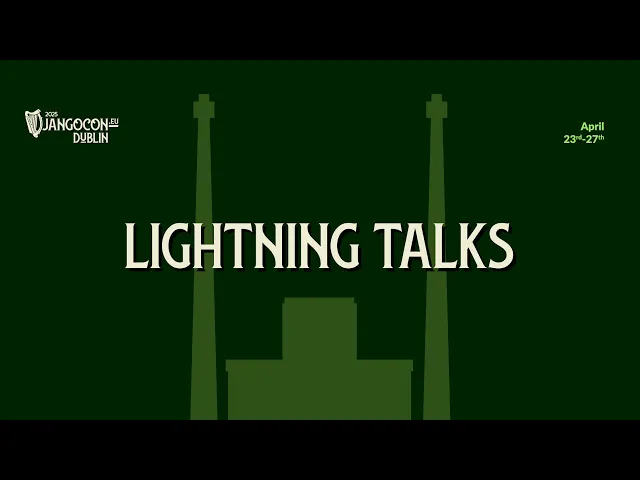
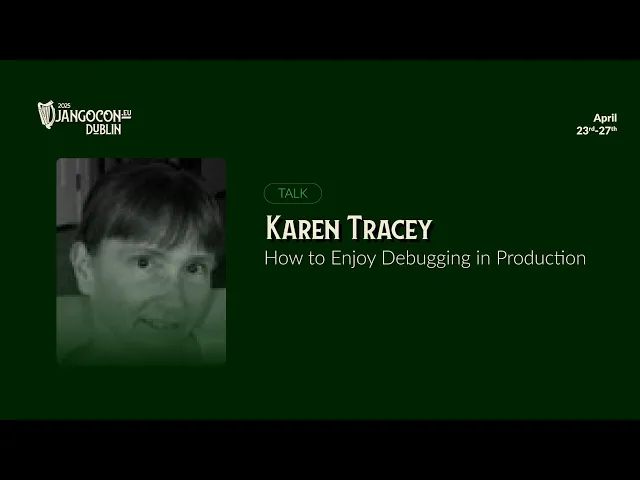
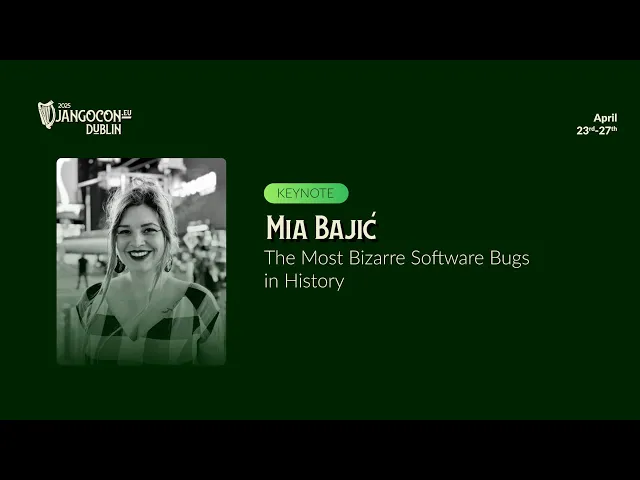
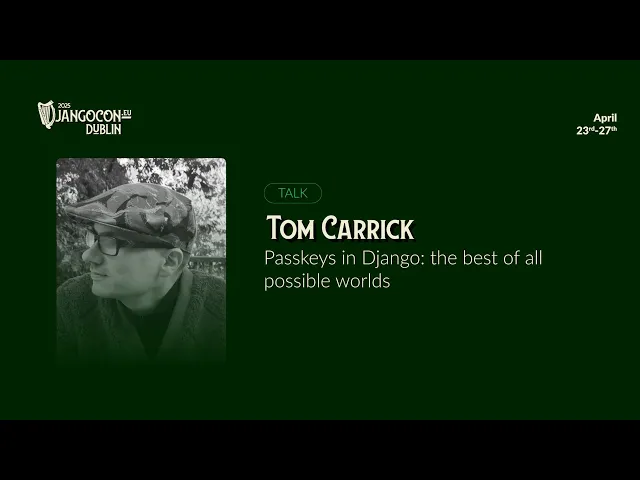
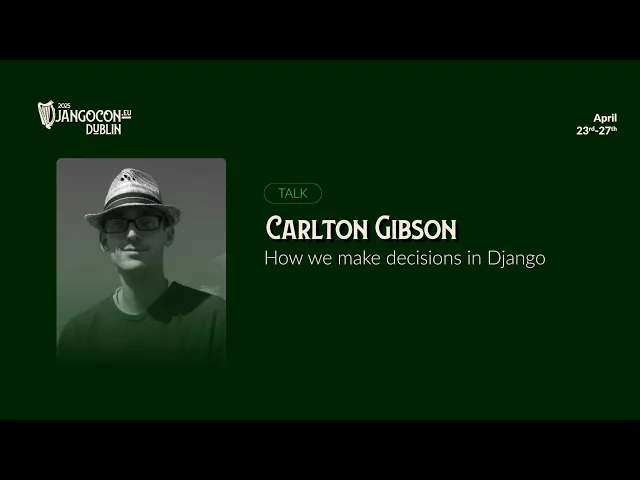
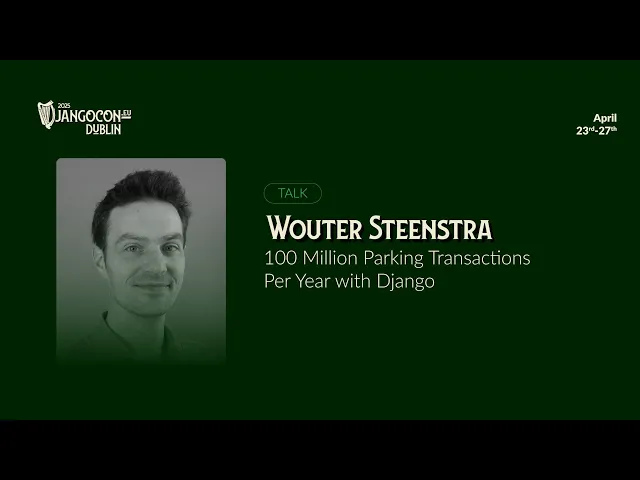
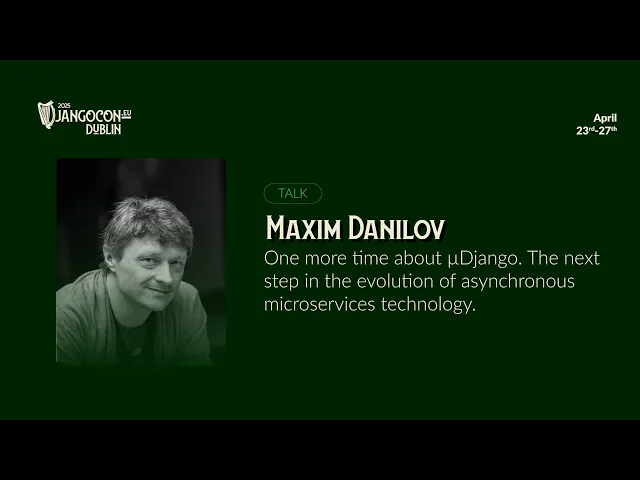
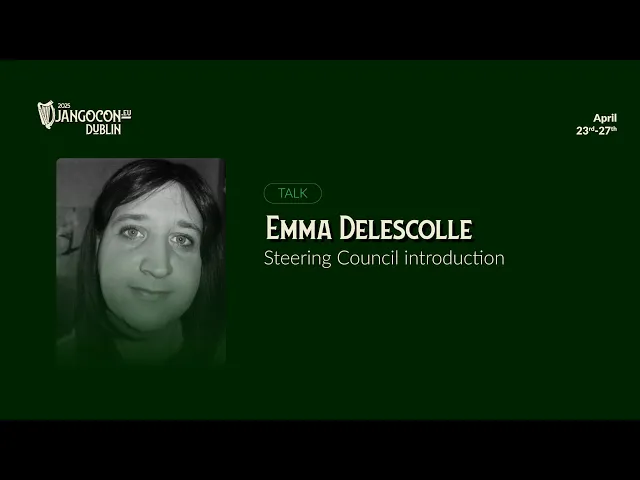
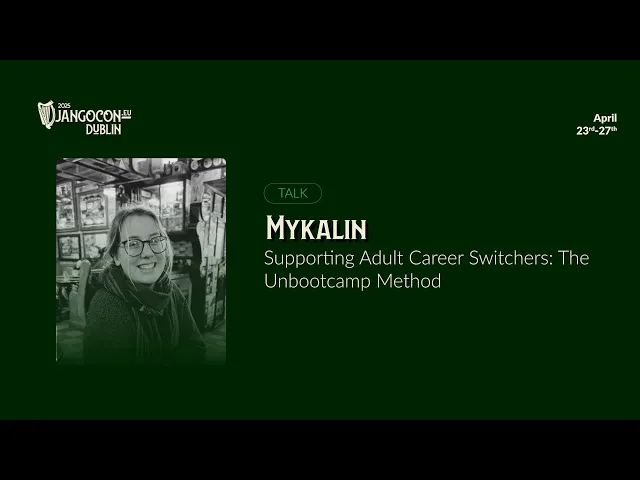
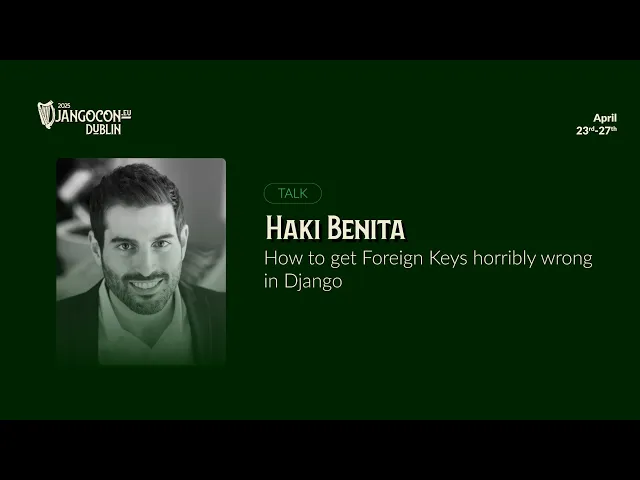
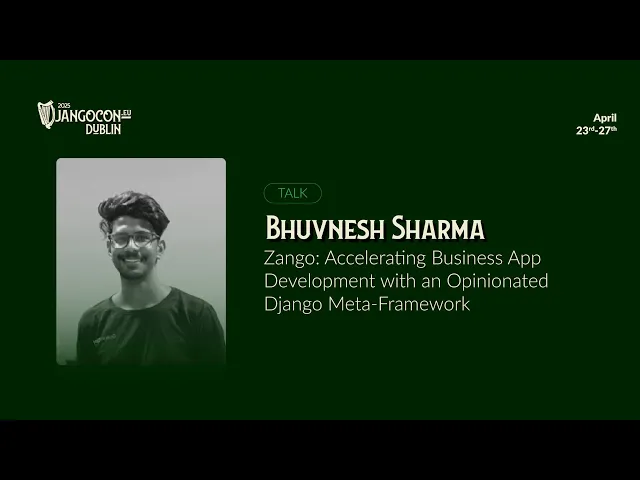
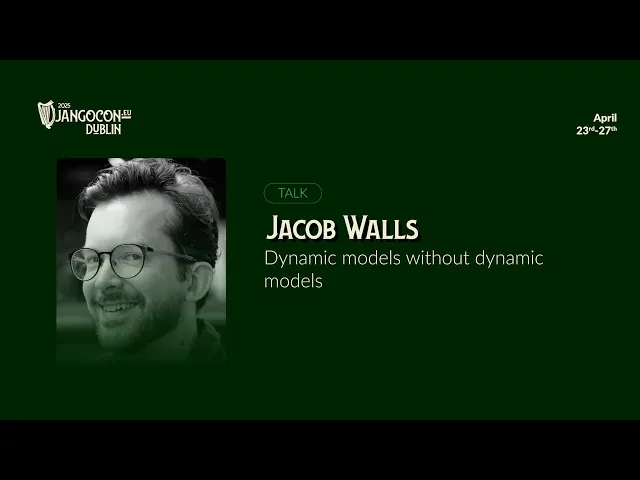
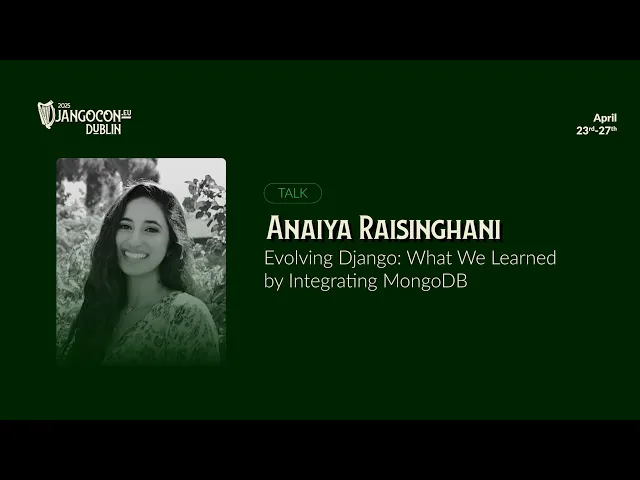
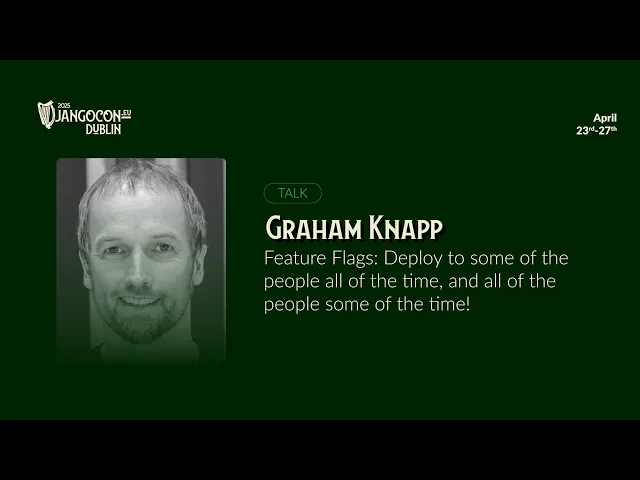
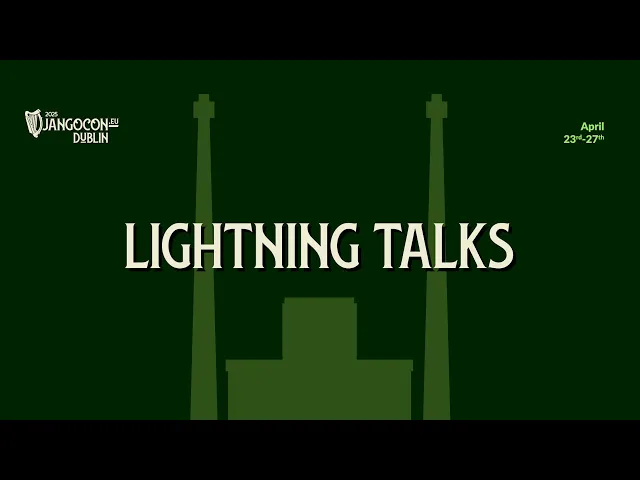
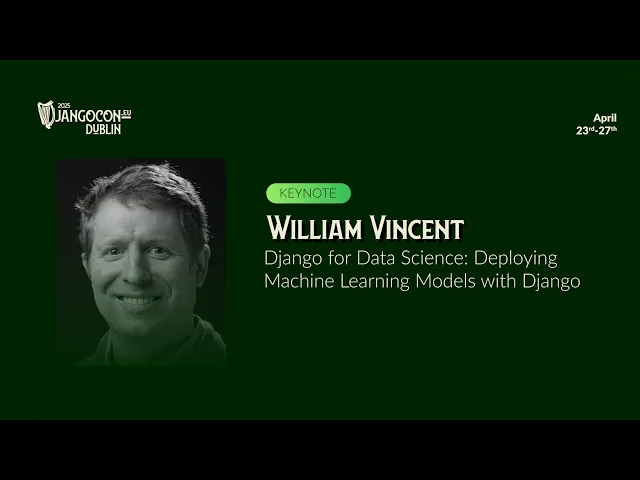
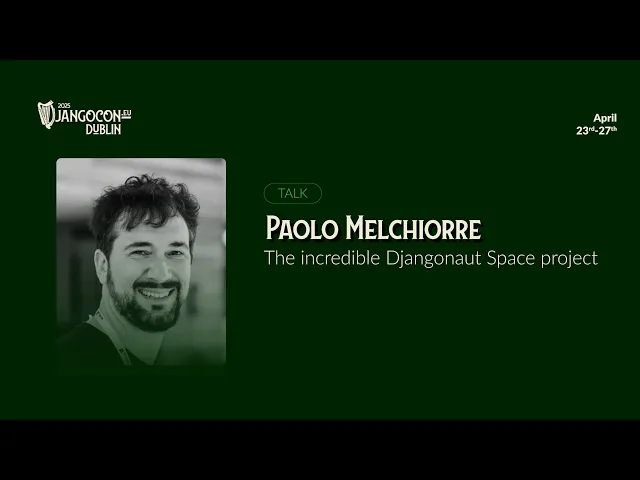
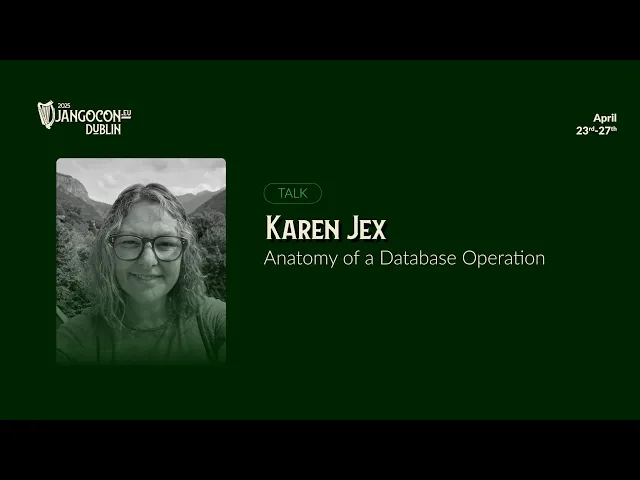
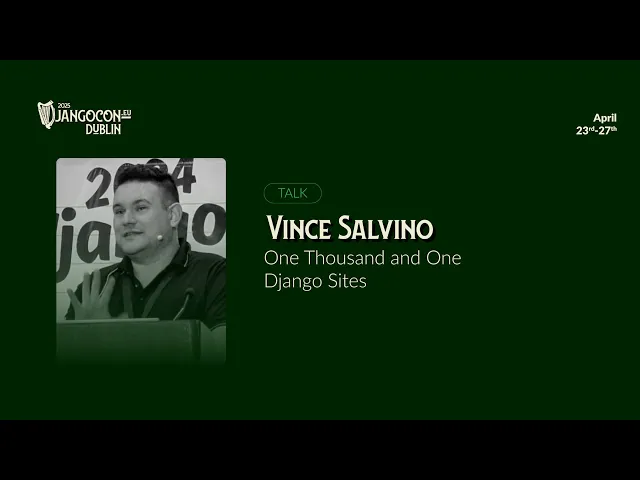
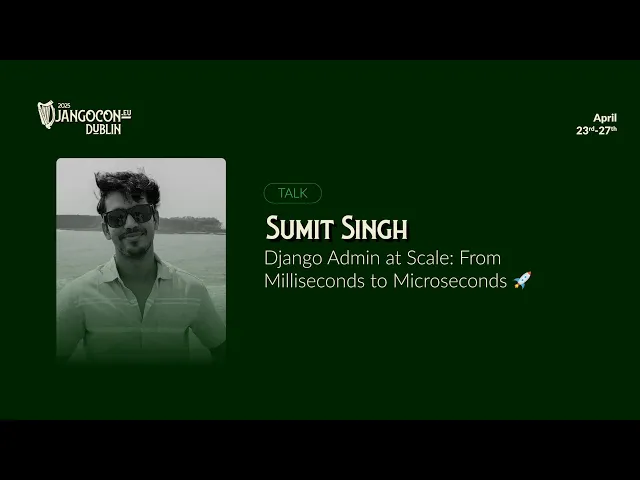
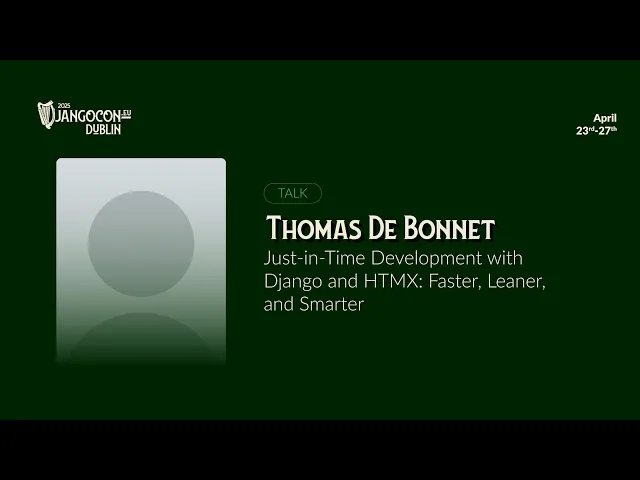
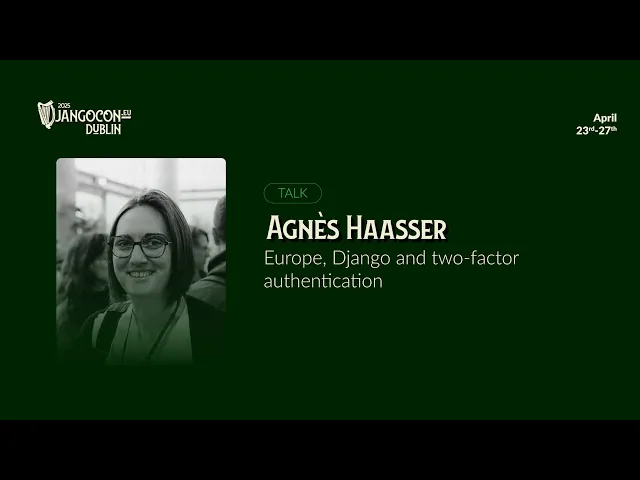
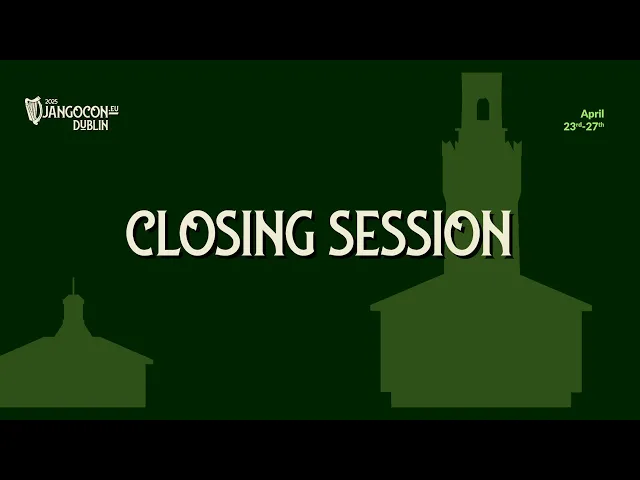
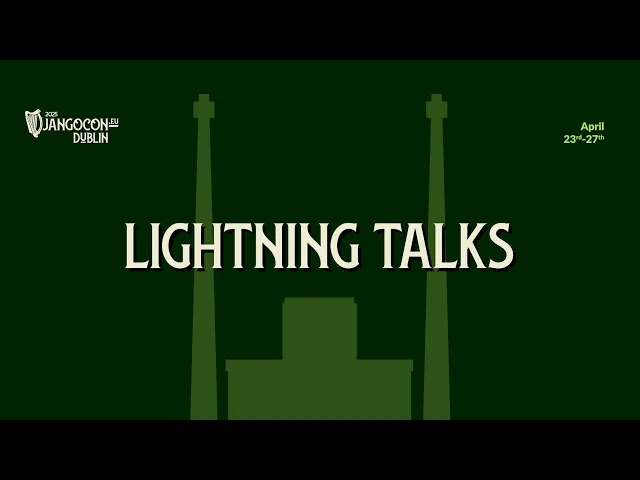
June 25, 2025
TestDriven.io
Building a Multi-tenant App with Django
This tutorial looks at how to implement multi-tenancy in Django.
Peter Bengtsson
Native connection pooling in Django 5 with PostgreSQL
Enabling native connection pooling in Django 5 gives me a 5.4x speedup.
Real Python
Your Guide to the Python print() Function
Learn how Python's print() function works, avoid common pitfalls, and explore powerful alternatives and hidden features that can improve your code.
Mike Driscoll
An Intro to ty – The Extremely Fast Python type checker
Ty is a brand new, extremely fast Python type checker written in Rust from the fine folks at Astral, the makers of Ruff. Ty is in preview and is not ready for production use, but you can still try it out on your code base to see how it compares to Mypy or other popular […]
The post An Intro to ty – The Extremely Fast Python type checker appeared first on Mouse Vs Python.
Real Python
Quiz: The Python print() Function
In this quiz, you'll test your understanding of Python's built-in print() function, covering how to format output, specify custom separators, and more.
PyPodcats
Trailer: Episode 9 With Tamara Atanasoska
A preview of our chat with Tamara Atanasoska. Watch the full episode on June 27, 2025
Talk Python to Me
#511: From Notebooks to Production Data Science Systems
If you're doing data science and have mostly spent your time doing exploratory or just local development, this could be the episode for you. We are joined by Catherine Nelson to discuss techniques and tools to move your data science game from local notebooks to full-on production workflows.
June 24, 2025
PyCoder’s Weekly
Issue #687: Scaling With Kubernetes, Substrings, Big-O, and More (June 24, 2025)
Real Python
Starting With DuckDB and Python
Learn how to use DuckDB in Python to query large datasets with SQL or its Python API, handle files like Parquet or CSV, and integrate with pandas or Polars.
June 23, 2025
Real Python
Python enumerate(): Simplify Loops That Need Counters
Learn how to simplify your loops with Python’s enumerate(). This tutorial shows you how to pair items with their index cleanly and effectively using real-world examples.